搭建训练的环境参考yolo的官网:https://github.com/ultralytics/yolov5
获取工程可以从yolo的官方网站获取或者直接从跟本人的网盘获取(非免费介意误下载):无需配置训练的数据解和下载数据,搭建好训练环境后就可以使用本工程直接训练路面积雪的检测:链接: https://pan.baidu.com/s/1lN4xoiLmqkOjNrip8R4XOg 提取码: ir1a ( 内部包含修改后的工程和训练用的数据集:训练855样本测试65样本,训练好的模型可以直接使用哦 )
准备好数据后配置文件coco.yaml
# class names
names: ['snow cover']
# number of classes
nc: 1
# train and val data as 1) directory: path/images/, 2) file: path/images.txt, or 3) list: [path1/images/, path2/images/]
train: classself/coco/train2017.txt # 118287 images
val: classself/coco/val2017.txt # 5000 images
test: classself/coco/test-dev2017.txt # 20288 of 40670 images, submit to https://competitions.codalab.org/competitions/20794
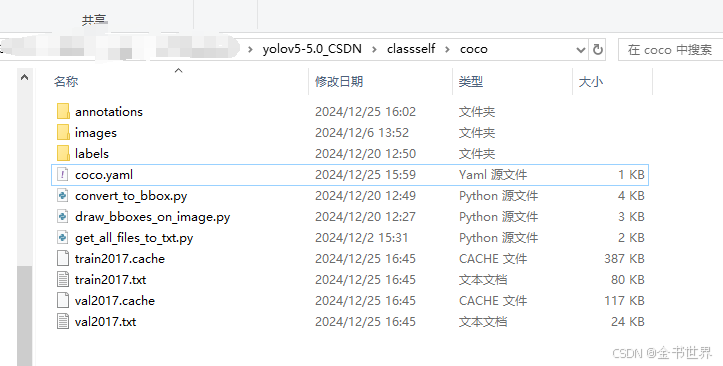
准备好数据后配置文件yolov5s_self.yaml
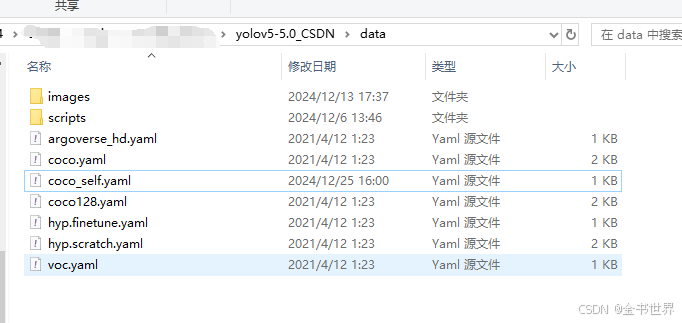
开始训练
#训练
python train.py --data classself/coco/coco.yaml --weights '' --cfg models/yolov5s_self.yaml --img 640 --workers 0 --device 0
#断点续训
python train.py --resume runs/exp/weights/last.pt
python train.py --resume
训练模型保存路径
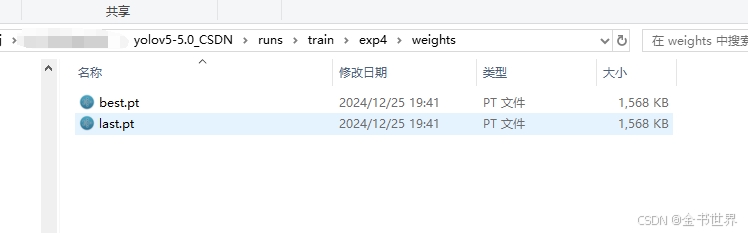
测试
python detect.py --source classself/coco/images/val2017 --weights runs/train/exp4/weights/best.pt
测试结果路径
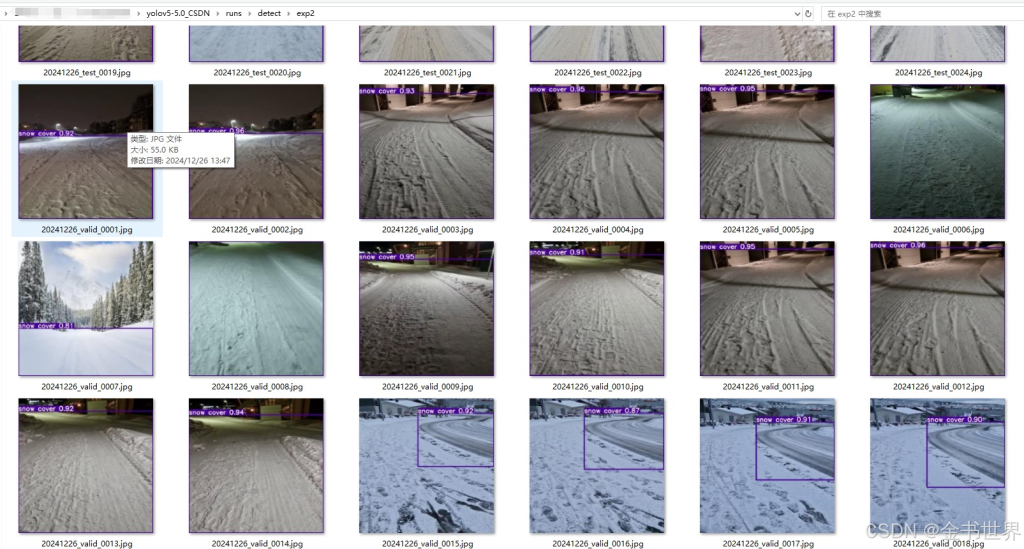
测试结果:
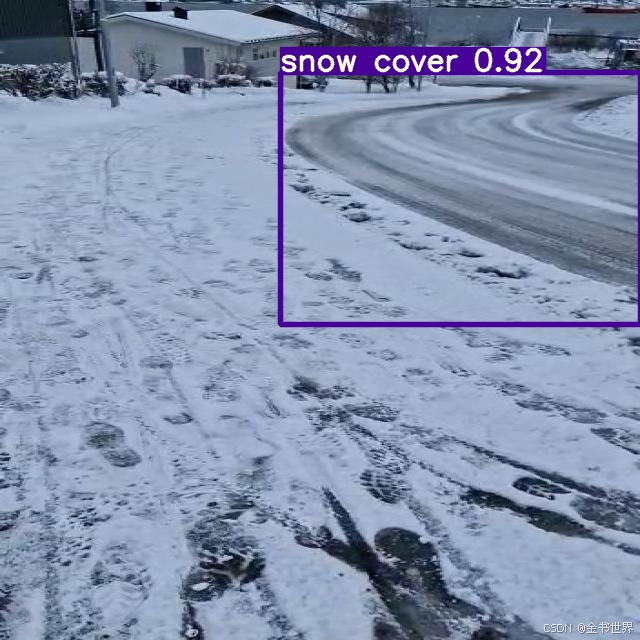
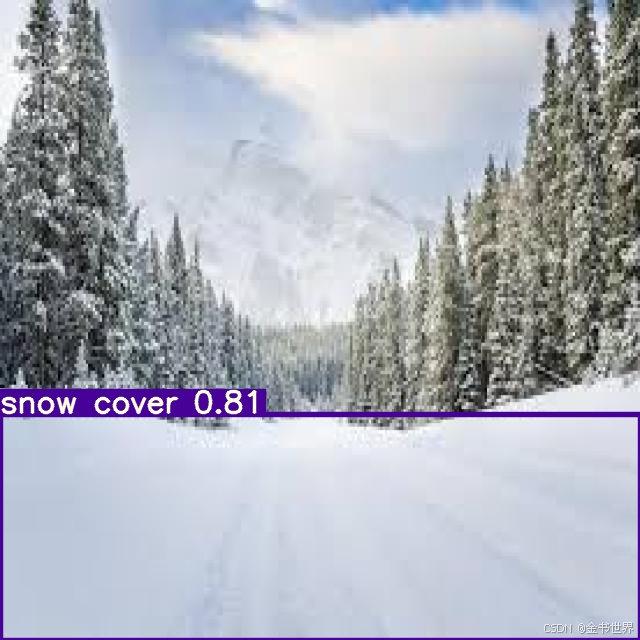
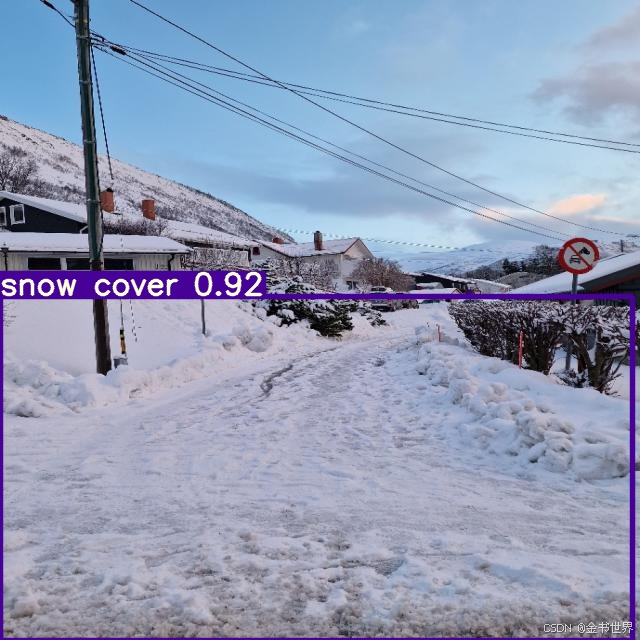
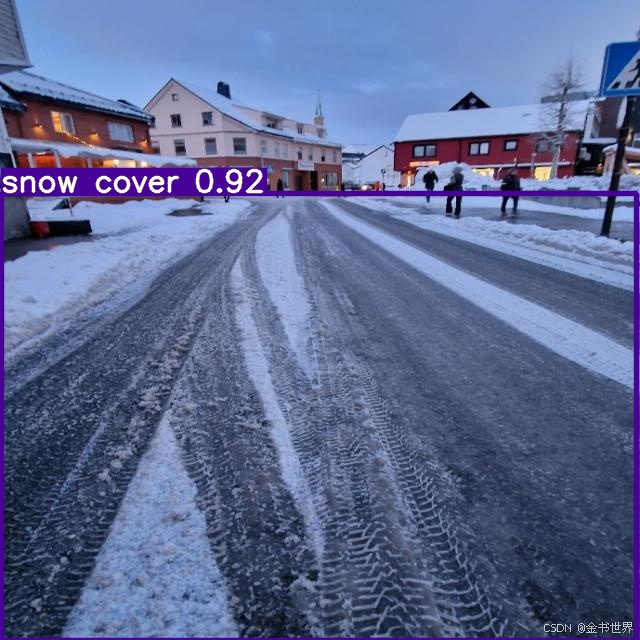
-=||=-赞 (5)